Explore Voyantis
Our team will contact you to schedule a call about your marketing needs and goals. We'll work together to find a personalized solution that fits your needs.
Redirecting you to our Case Study Hub in 3 seconds or click here
Value Based
Eliminate uncertainty in growth - our Predictive AI reveals each customer’s true value, powers value-based campaigns on Google and Meta to acquire high-value users, and automates timely actions to activate, nurture, and monetize them.
Does this sound familiar to you?
What if you knew which customers are worth your time and how to convert them?
With AI we identify high-value customers and sync actions with acquisition platforms like Google and Meta, or engagement partners like Braze and Salesforce.

Acquire
Boost your ad network campaigns with Voyantis’ predictive signals and automation. Our AI analyzes non-PII data, such as engagement and spending patterns, to identify high-value customers and syncs pLTV data with your ad network, enabling tROAS or Value-Based Bidding campaigns to attract similar users.
Activate
Optimize your time and budget by skipping low-value customers. With Voyantis AI, identify users most likely to pay, stay, and upgrade - and discover the best offer to convert them.
Monetize
Maximize your customer base's value. Our AI identifies accounts ready for upsell, expansion, or cross-sell opportunities at the optimal moment, delivering personalized recommendations to drive success.
Retain
Improve retention with our AI. By identifying at-risk users through signals like technical issues and decreased usage, our AI assigns a risk score - enabling timely, targeted actions to rebuild trust.
Take action with your acquisition or engagement partners.
How it works.
Transforming past data into future-proof actions.
Only non-PII data.
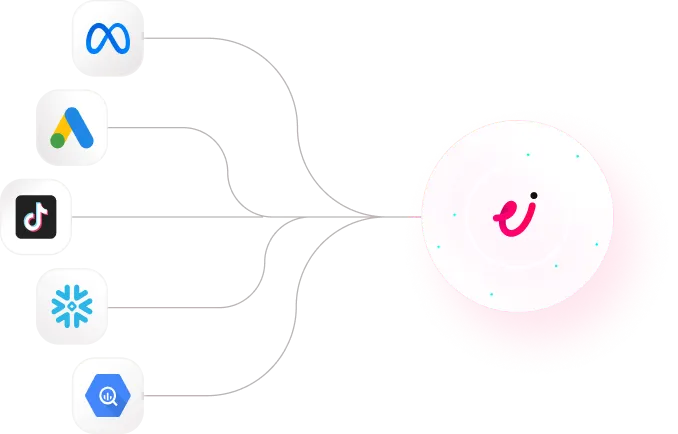
We integrate with data sources like Snowflake, AWS, and BigQuery to access non-PII data - device, location, interactions, declarative and more - powering user-level predictions from Day 1, refreshed hourly. AND we are ISO 20022 and SOC type 2 certified.
Robust predictive modeling.
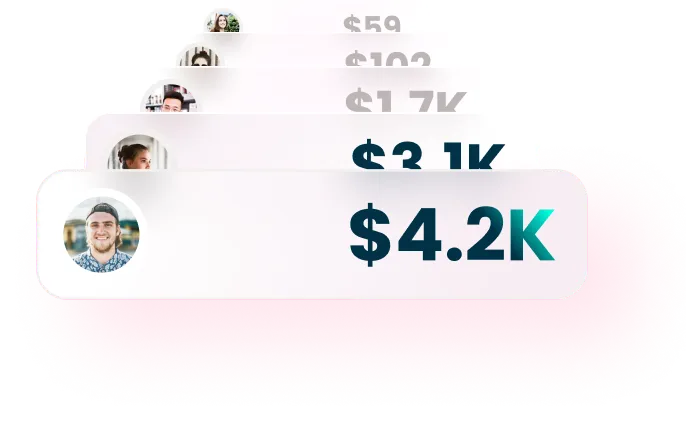
Our AI aligns with your core business objectives, analyzing historical customer behavior to build bespoke models that predict lifetime value, spend, and behavioral patterns at the user level. This spans the entire customer lifecycle - from acquisition and activation to retention.
Knowing how to interact with ad networks.
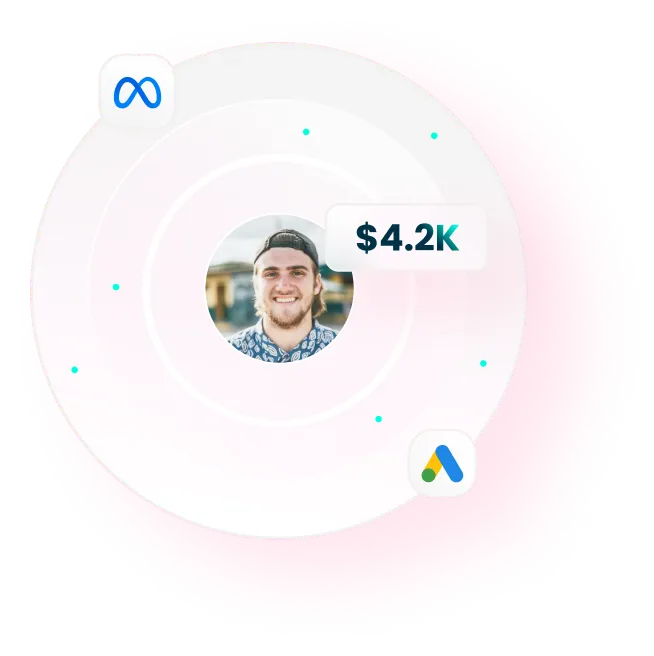
With predictive LTV you can move to tROAS campaigns. But predictions are just the beginning. Success lies in a smart signal strategy - automating delivery with the right timing, accuracy, and value while adapting to each network’s quirks. Every signal is optimized for maximum impact with our Prescriptive AI.
Connecting with your engagement platforms.
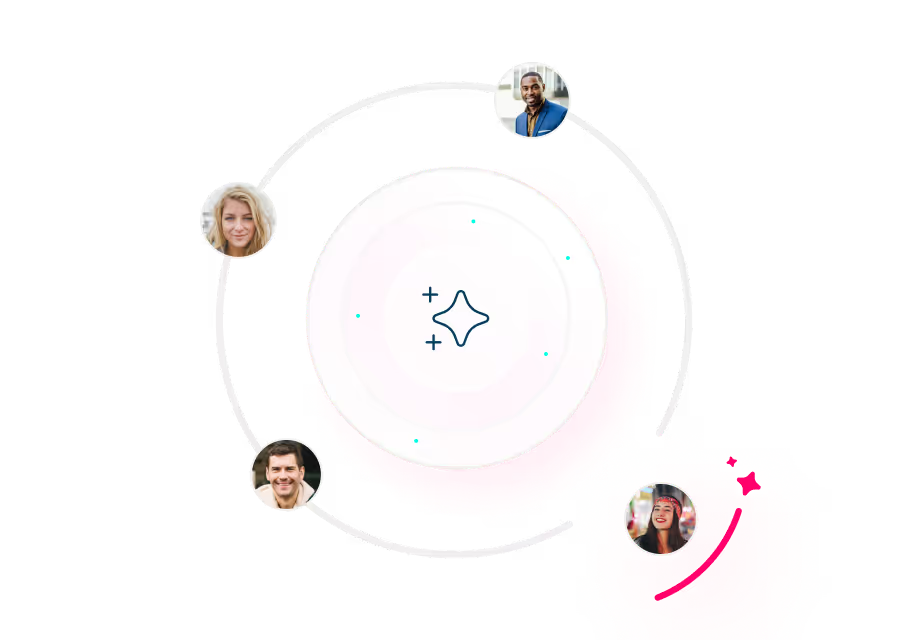
After acquiring a user, the next step is converting them to paid and retaining them. Our AI uses value-based segmentation to deliver personalized actions, driving engagement, spending, and retention - all automated through platforms like Braze.
Always-on optimization.

Voyantis continuously monitors your models and data to ensure that data drift, outages, or anomalies don’t disrupt your campaigns or lifecycle optimization efforts. We also collaborate with Meta and Google to keep our models aligned with theirs, helping you stay ahead. The result? Significant cost savings, effective risk management, and substantial performance gains.
Pre-built connectors for seamless integrations

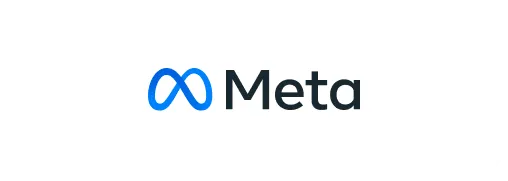







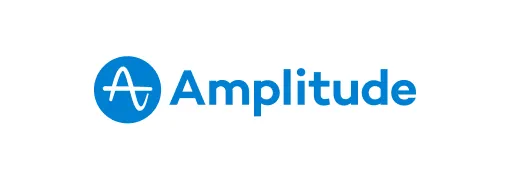


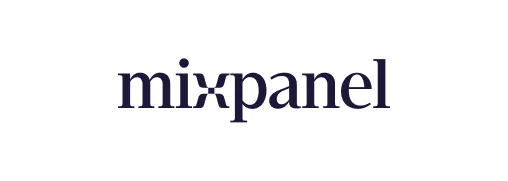



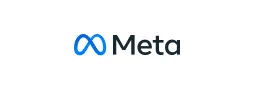
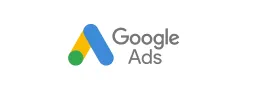



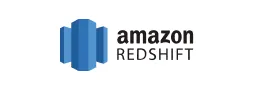
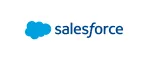

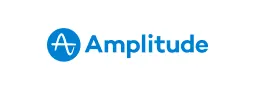

Accredited by the international standard for information security
With each customer’s LTV in hand, all your growth metrics will soar.
You can achieve these solid numbers too.
What’s Next
FAQ
Does Voyantis use PII data in creating predictive models?
Voyantis develops bespoke prediction models using strictly anonymized data such as fully anonymized engagement telemetry, transaction, quizzes, and onboarding inputs.
What is LTV prediction?
LTV Prediction is a method to accurately estimate the total revenue of a customer during their interaction with a company. In order to generate an accurate prediction, a model should integrate historical data including churn, retention, and revenue and to be able to model the behavior of different customers. Once accurate models were trained and tested, they could be used for prediction of LTV of new customers/ads/campaigns, etc…
How do you calculate predictive LTV?
Building an accurate model to calculate the LTV of a specific customer is not a simple task since there are many factors that should be taken into consideration. It is recommended to factor into the model historical data customers from their first purchase and throughout their interaction with the company and to compare it to the behavior of the specific customer that requires the prediction. It is recommended to use statistical tools or machine learning models based on AI to represent these models and to test the models for accuracy using historical data.