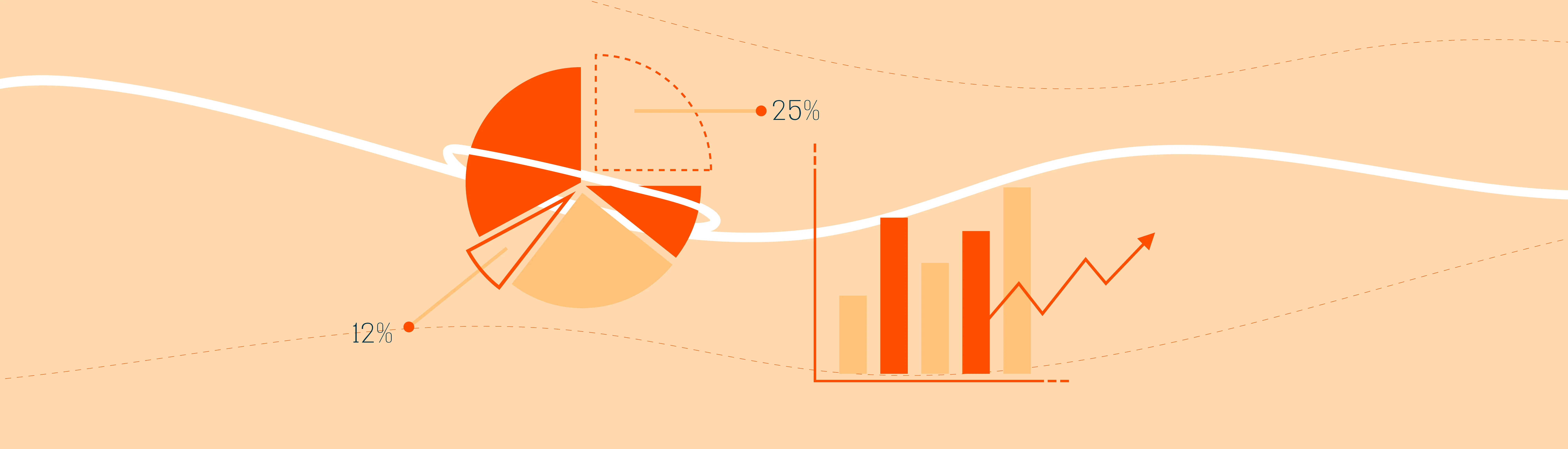
Tips and tricks to build the best data team (your growth team will thank you!)
Privacy limited restrictions are making it clear that companies need to invest in data infrastructure. Even companies that didn't have such infrastructure in the past are now beginning to explore ways to build an in-house data team. After all, having access to raw data is becoming more important to companies that didn't invest in that previously, which is especially the case for DTC brands. Building this infrastructure is hardly an easy undertaking.
From our experience (both on the front lines, and after talking with dozens of growth marketers,) instead of solely focusing on the short-term, you would need to think long-term, as well as how to later handle scale, LTV, retargeting. In fact, even in the initial stages, it is best to plan for years ahead. The most robust data structure will support advanced uses of data, such as data modeling and predictive modeling. In turn, that will make the data structure able to support the growth team in a manner that facilitates the transformation of a mass-market approach, to a personalized approach. Of course, there is more to that than reaching target audiences with relevant messaging at the right time and point of the customer journey—it also plays a role in the optimization of budgets and spend for each segment of users being targeted.
The best, surefire growth marketing decisions are the ones that are based on data analysis. Data insights amplify user acquisition and ROI in a measurable way, and can easily be seen through the improved performance when targeting and optimization goals are based on historical data.
There’s much more to consider when building a data team, especially when considering their future collaborations with the growth team. For instance, what are the strategies that your brand would need to take on, based on maturity? What are the unique skill sets your analysts will need to accomplish goals pertaining to growth and retention? And of course, what are the tools and solutions the data team would need, to activate the superpowers needed to help maximize results for the growth team?
I know, it sounds like a lot. The good news is that approaching this can be easier done, than said.
Let’s take baby steps, shall we?
Starting with the right perspective
The first step to building a strong data team tends to be hardest, as there is a chicken-or-egg type of conundrum: Would it be better to first hire a data engineer to build technical infrastructure, or would it make more sense to hire an analyst to figure out what data is needed and use that knowledge to determine what that infrastructure should look like?
Naturally, that depends on the industry, product, and service at hand. However, it’s important to take a step back and realize that data itself should not be viewed as a team to hire or a set of tools that should be implemented. According to Reforge, it should rather be seen as a strategic lever for growth.
Think beyond the team and tools
Data-related issues are often rooted in areas that have nothing to do with the engineers at hand. For instance, it is possible that the data capabilities don’t align with the strategy and goals of the brand. It is essential to ensure that the product strategy is well-defined, and that the data capabilities can cater to the strategy accordingly.
In the case of one of our clients (a DTC subscription brand), their data lake does not hold information about order-level COGS (shipment cost, inventory costs, taxes, etc). It is therefore very hard to calculate user level predicted gross profit. They only get the average margin from their financial department, as one number per product for the last quarter.
And here’s another example... A hardware device that provides a SaaS over that device (fitbit-like, for example) is marketed and sold digitally. That’s great, but a signup only happens after getting the device, and no attribution connects the sale of the physical device to the subscription signup, yet it's a simple matter of using a QR code, or sending an activation code at time of purchase of the physical device. No amount of data scientists would be able to provide a decent prediction on the LTV of a device that was purchased, not to mention ROI/ROAS predictions
Data-related issues also tie in with the experience of the data analysts at hand. It is ideal for their previous experience in working with data to align with the current stage that the brand is in. After all, an analyst that is used to scanning the data of early-stage companies would suddenly be thrown into the lion's den if tasked to go through mature company data. This leads to a series of misapplications, and oversights that can snowball into undesirable outcomes when it comes to user acquisition efforts.
Building out the best, all-star data team calls for more than just hiring a bunch of skilled analysts that appear to be at the top of their game. There are some areas (which we’ll delve into in the next section) that need to be considered and covered, in order to ensure all the boxes are ticked to enable data as a lever for growth.
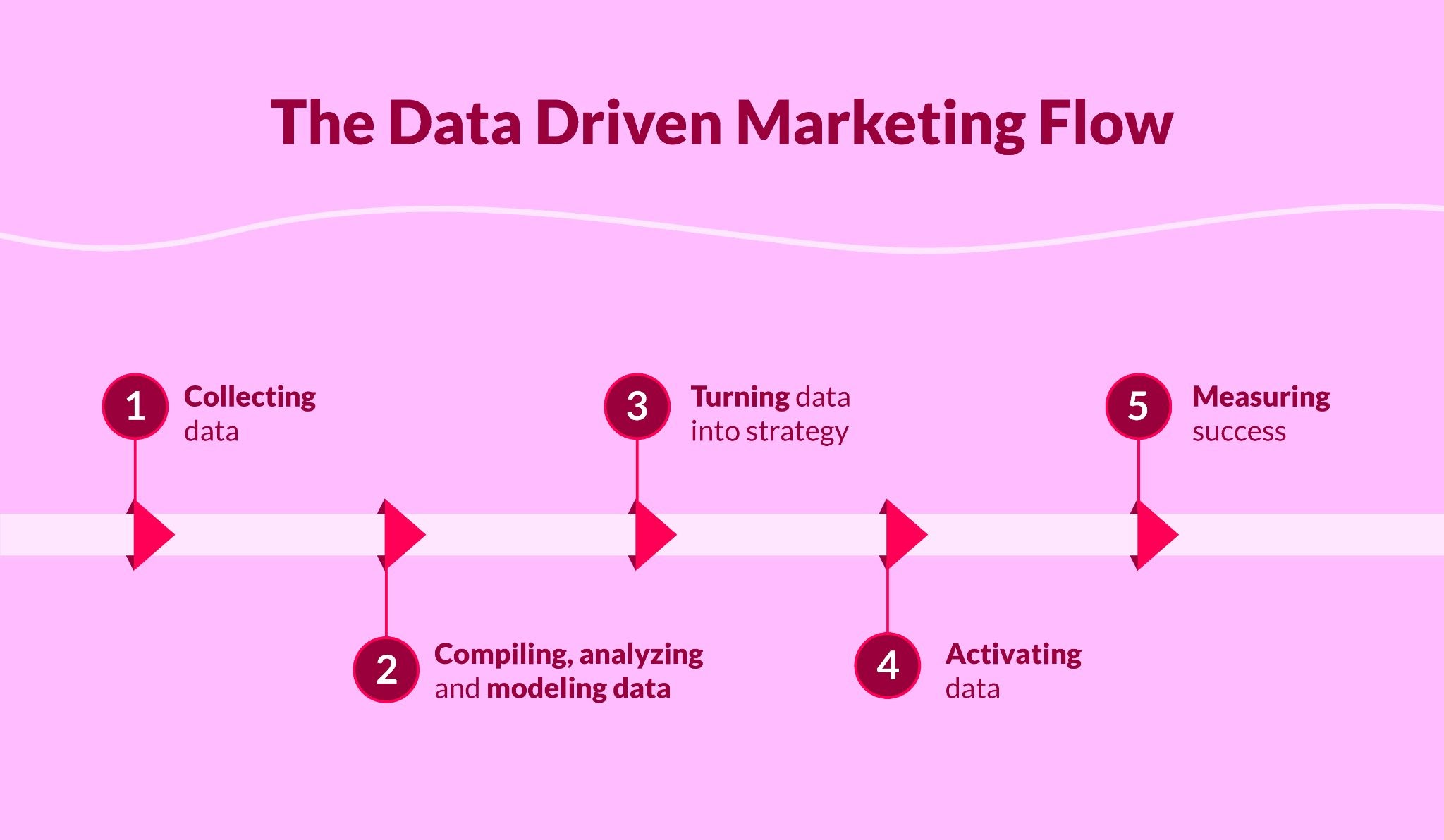
Data as a lever for growth
The following are the four main areas that teams would need to consider when building out their data capabilities:
Strategy
What are your points of leverage? How does data improve those points of leverage? After all, the answer to questions like “when do I need a data scientist?” really starts with an objective reflection of the brand's strategy, roadmap, and goals. For instance, your quiz data and survey data is extremely valuable, and it is recommended to get it stored as raw data (and make sure it is well connected (attributed) to both the marketing efforts and the future transaction data), to enable running future analyses when needed. The utilization of such data points can help ensure that customers are addressed in a more personalized manner, which significantly improves the customer experience.
Stage
For more mature companies, there are countless possible sources that data can be taken from and the volume of data is enormous. This is especially because new contact points between companies and customers emerge constantly. New information is also generated at each stage of the customer journey: online and offline, mobile and social, locally and through market research.
What stage of maturity is your product in? What stage of maturity is your data in?
This is needed because the realization of this opportunity cost is painful, and making up for it can take hours of realigning metrics definitions, sourcing available data, backfilling data pipelines, and possibly even a realignment of the company’s culture. Ideally, there also shouldn’t be departmental silos, because the success of data-driven marketing is largely dependent on having high-quality and integrated data. When there are silos in place, different departments will acquire data with goals that contradict one another.
Team
What types of people do you need to achieve the data strategy? Analytical thinkers? People with software-specific skills? Experts in data visualization? Will the team be equipped with the setup for success internally early on? This isn’t just about having the right heads in place, but about making sure that the org is working well together by collaborating. This may call for devising a skills development plan for the team, to ensure they are proficient in modern data stacks.
Tools
What tools do you need to adopt to facilitate the team's impact? There needs to be user level reporting capabilities for UA efforts. This can be accomplished building your own BI, or using a tool that supports row data exporting from the get-go such as GA4.
External tools are excellent to fill data-related gaps, especially if they are plug-and-play, as that saves companies time and resources from building solutions in house.
The ability to send LTV signals is made easier when using a UA predictive solution, but there is much that needs to be considered before implementing a tool that will not only send relevant user-level optimization signals to the ad networks (bypassing the current iOS14.5+ issues) but also reach new standards of optimization. This is also important when considering the changes in all networks and MMPs— one would want to make sure they have all of the raw data at the user and session level. External tools can be used for advanced functionalities once you have the basis built the right way. With that in mind, we put together this list of nine questions to use when implementing a UA predictive solution, in order to understand which is the right one that fits the team, budget, scale, internal resources and more.
All in all, it is important for teams to keep revisiting their data management strategy and evaluate how well the team and all technology solutions are working. As you build, you will want to make changes and bring in new tools to make improvements.
Subscribe for more
Read expert stories, interviews, reports, insights and tips for profitable growth.