.webp)
Do growth marketers really need a boost to amplify UA campaigns on FB and Google? (Yes!)
We have to give Facebook and Google a lot of credit. They got a lot of things right in their ad networks. Of course, it shouldn’t come as a surprise, considering the fact that they invested heavily in machine learning capabilities to drive traffic such that they maximize the value stemming from user acquisition campaigns.
Yes, the campaigns do cost a pretty penny, and it is a two-way street. The yield that comes from creatives, and a target “conversions” is impressive when it comes to short term conversions. But that’s also where the blind spot of the ad networks come in. They are not optimized for the long term. The true behavior and value of users does not manifest immediately, and definitely not with a single signal.
Ad networks essentially lay it on the growth marketers to point out, or model, what a truly “valuable user” is, and do so very quickly so they can iterate and optimize their own machine learning. In the case of Facebook, this needs to be within seven days from user acquisition. Google allows for up to 90 days, but is known to not perform as well.
This is why growth marketers need a boost to amplify their UA campaigns on ad networks such as Facebook and Google.
Growth marketers can better understand the behavior of high value users through reverse analysis. What this means is—instead of spending time to come up with different ways to target new users, it’s better to zero in on what existing users are responding to across the board, and work your way up from there.
If you decide to do some reverse engineering on Facebook ads, for example, you have the option to upload a list of your top users as a “Custom Audience,” use the “Audience Insights” tool to learn more about your customers on Facebook, and utilize that information when configuring target audiences for your Facebook ads.
Of course, Facebook isn’t the only source for brands to gain insights on users. If anything, growth marketers know all too well that the most insightful data on audience behavior is right under their noses—on their own platform. One can further delve into rich data using business intelligence tools, and marketing analytics platforms, such as GA4, to gain rich insights on events such as app/screen/page views, button clicks, system events, user actions, transactions, and more.
The best way to go about conducting a reverse analysis in general is by locking a segment of high value users, and analysing their meaningful behavior indicators.
While doing this, we recommend paying attention to the tension between the size of the segment, to how specific it is. Ideally, you would want it to be specific on one hand (per location, per acquisition channel, etc), but you also wouldn’t want to end up with a limited segment that is too small. The segments can also reflect purchasing behavior of users. For instance, when do they make their purchases? What do they purchase? Do they make multiple purchases over time?
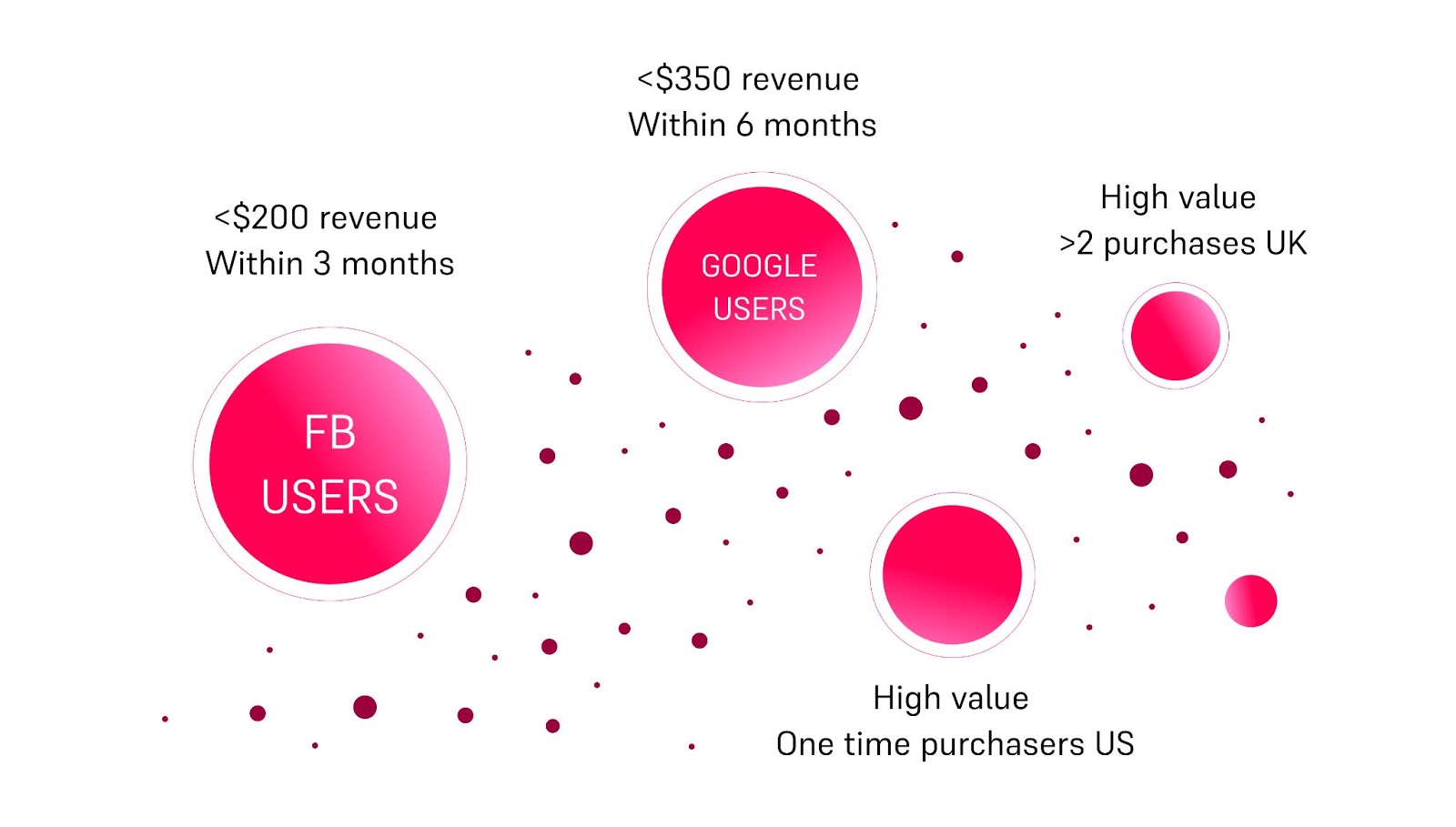
Upon acquiring insights on high indicative behaviors, the next step is to leverage them to send signals to ad networks. A Server Side conversion can be used for this.
For brands that have an in-house data team, or an internal LTV model— growth marketers can leverage the insights/indicators from the model to send the enhanced conversion signals to the ad networks. Leveraging those insights enables the marketer to optimize conversion events according to the signals, and also push users during the trial stage to complete the stage.
With AI, predicting LTV won’t take a lifetime
When backed by AI, user-level LTV predictions can look and optimize as far ahead as you’d like, whether it’s a matter of a few weeks, or even a few years. In our case, Voyantis’ UA suite is composed of several products, which, when combined, offer 360 degrees of evaluation and optimization capabilities. The business value of UA campaigns with LTV signals can be seen within a month.
We provide our clients with a full accounting of the expected rollout of value over time. Of course, we depend heavily on the amount of first party data (engagements that the users have had with the brand) that our clients have available to-date, and enrich it with our third party daya. The single user prediction is then used as a signal optimization value to the networks, allowing brands to create lookalike campaigns at scale.
We elaborated on how this was achieved for a casual gaming company in this post, and how we did it for a subscription goods brand in this post.
Significant returns from the added boost of growth superpowers
When growth marketers and user acquisition managers make use of LTV signal optimization, they can experience significant performance improvements over their go-to UA strategy:

If you think about it, the results that come from optimizing UA campaigns based on LTV predictions offers a wealth of marketing value for business owners and their brands, by directly improving marketing KPIs.
All for one, and one for all!
Can such a solution be developed internally?
There are many iconic brands (with deep pockets) that have invested heavily in building internal predictive UA optimization capabilities. We recently wrote about how Lyft did so with their very own “Symphony.” As you can imagine, creating such a solution calls for endless engineering, devops, and project management hours. That is in addition to budget, and consultations with experts on UA and growth.
Brands are better off focusing on their business and customers, and there is no time like the present for growth marketers and user acquisition managers to capitalize on the power and potential of AI, and LTV signal optimization. At this point, while competition is fierce across ad networks—brands can’t afford not taking the extra step to reach the full potential of their UA campaigns.
Subscribe for more
Read expert stories, interviews, reports, insights and tips for profitable growth.