
Growth and ROAS is serious business for gaming companies
The gaming realm has seen significant growth in the past decade. The market value of the industry went from an already-impressive $52.8B in 2012, to $138.4B in 2021, and the only way to go is up. However, there is one barrier that is preventing gaming companies from reaching their full potential—the UA limitations brought on by ad networks. Thankfully, there are ways to workaround these limitations, and that is through a UA optimization solution that makes use of LTV predictive models.
Casual gaming companies are not reaching their full potential
Casual gaming brands typically optimize their UA on Facebook’s ad network by focusing on a mixture of campaigns, such as installs, and purchases that occur between the standard one to seven day conversion window. That might sound harmless in theory, but this brief window doesn’t allow campaigns to target their full potential. It creates fierce competition between other publishers in the space, and the casual gaming companies themselves end up missing out on a significant portion of their potential audience and revenue.
One of our own use cases
We speak from experience. We recently helped a casual gaming brand uplift their ROAS by 150 percent. This is a brand with seven-digit spend per month, primarily on Facebook, with an expected payback period of 8-9 months. This company wanted to expand their audience beyond the CPI ceiling, and this was only possible by creating a single predictive metric to target their long-term LTV audience, lower their CAC, and increase LTV and profitability at scale. Of course, this is easier said than done. However, it is entirely possible by tapping into the power and potential of AI.
An LTV prediction model (based on an internal data lake) was created, and activated by sending a custom conversion signal to Facebook, allowing the company to run the campaign by optimizing on the signal.
The results (stemming from predictively looking well beyond seven days) speak for themselves.

Those are some impressive results, and you might be wondering how we managed to get there. It was a simple 4-part process.
- We started by meeting with the brand to set the prediction modeling goal, which was high LTV late bloomers in this case, which refers to users that would purchase late and are predicted to yield high value. It was determined that this would be the best goal, upon analysis of their needs and current user behavior.
- The next step was to build the prediction model, which took less than an hour of R&D on the customer side. Once that was established, we automated the process of cleaning and normalizing the data to build the LTV model.
- Next came the “superpower” element—the ability to act seamlessly , and "capitalize" on our predictions, which enabled Facebook to find audiences with high LTV. This refers to people who would start purchasing outside of the limited conversion window. They are typically not reachable using standard targeting and optimization goals, but it becomes possible with the predictive model. This leverages the publishers' first party data into a single signal for Facebook.
- The brand went on to duplicate the best-in-class campaign, optimizing on the Voyantis Optimization Signal. This ran alongside the original campaign on a similar budget, which started at ~$50K and ramped up to ~$200K monthly.
The graph below compares the ROAS of late bloomers vs. early birds . The ROAS of late bloomer campaigns increases over time and in some cases may surpass that of early bird campaigns. (Note: the ROAS of early birds is higher during the conversion window, which can make late bloomer campaigns seem less effective to the untrained eye.)

Here’s a look at the numbers from the Voyantis campaign, versus the benchmark campaign. The data from the two campaigns is from two different points in time as it progressed—one after the first month, and the second after four months. You will see that despite the identical creatives and targeting, the difference in optimization from Voyantis’ signal led to far more favorable results across the board.
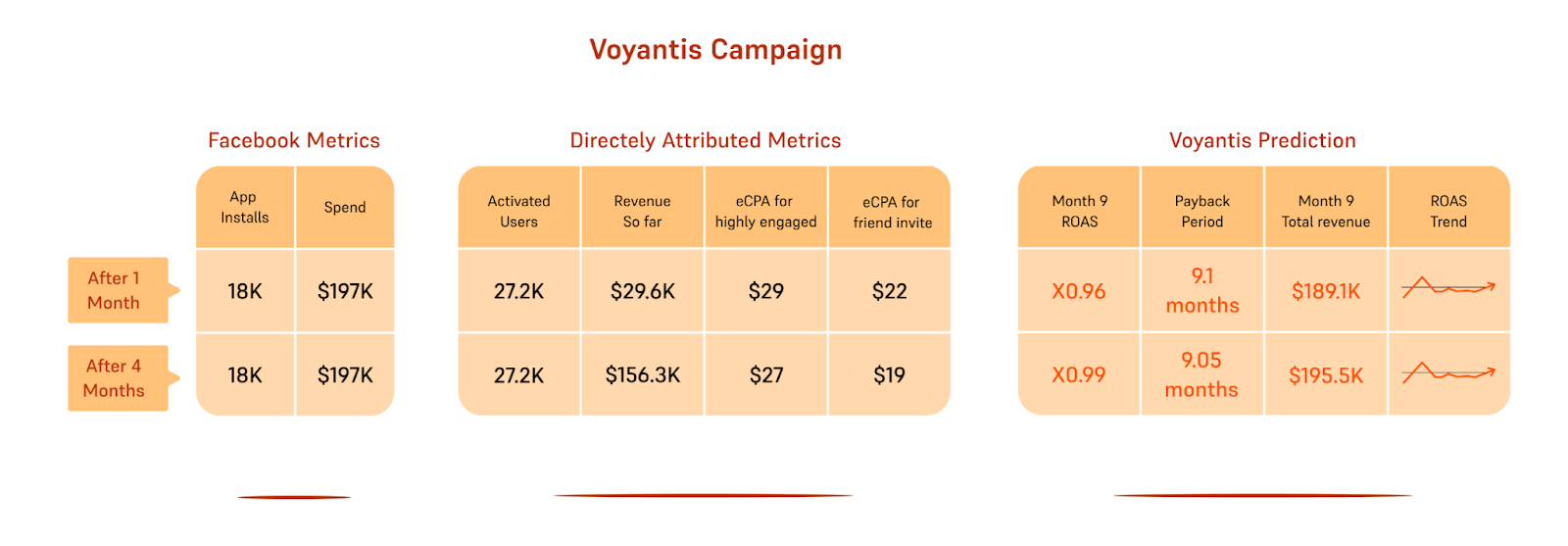

Marketers need a boost to optimize Facebook’s capabilities
Our use case (in which results were typical across gaming customers) proves that even the best of growth marketers with the deepest of pockets can benefit from an added boost to amplify their UA campaigns on Facebook. The ad network requires events to happen within a week, but the true LTV of users takes longer than that to calculate, especially in gaming. These are the users that have, and demonstrate, a higher user-product fit, and higher long-term retention.
LTV predictive models help marketers focus on users that aren’t necessarily early buyers, for higher long-term retention. It also helps them own their processes despite curveballs such as the IDFA changes in iOS 14.5. Predictive modeling can overcome obstacles by using a single signal to embody a user’s LTV based on a complete set of actions and behaviors, in addition to campaign performance. This allows marketers to send predictive signals for users who are most likely to make high-value purchases over time.
The time to amplify growth is now
According to a report by Accenture, the gaming industry has increased by half a billion players in the past three years, totaling 2.7 billion people globally. The report also states that more than 400 million new gamers are expected by the end of 2023. There’s hardly a need for competition to be fierce, and the market share is there for the picking. The best way to grab the lion's share of the cream of the crop is through the understanding, and optimization of user LTV through predictive modeling for serious growth and ROAS.
Subscribe for more
Read expert stories, interviews, reports, insights and tips for profitable growth.