
How to conduct a customer lifetime value analysis (using predictive marketing!)
“What’s the ROI of your mother?” Woah woah easy… not trying to start a fight! That’s a question famously asked by Gary Vaynerchuk to a conservative CMO, for some dramatic effect on the importance of engaging and nurturing customers.
Now on a similar note, let me spice things up and ask you—what’s the LTV of your customers? You see, unlike the ROI of your mother, which obviously cannot be measured, the LTV of your customers absolutely can!
In fact, once you obtain LTV data, which can (and should) be used in predictive models, and act on it—the sky's the limit. In turn, your ROI will reach astronomical heights in the long-term.
According to a report by ResearchAndMarkets, the global predictive analytics market size is expected to grow from USD 7.2 billion in 2020 to USD 21.5 billion by 2025, at a Compound Annual Growth Rate (CAGR) of 24.5 percent. With the exponential rise in organizational data, organizations are increasingly hiring teams of data scientists and analysts to help process and interpret collected data. This is also where predictive analytics solutions come into play, as it helps gauge the data that’s on hand, to help predict future trends across all fronts.

What are predictive analytics, and why is it important?
Predictive solutions analyze historical and real-time data about the decisions and behaviors of consumers and prospects to determine what gets them to make specific actions, such as purchases and upsizing.
The solutions and the data presented by predictive analytics exist to make life easier for marketing and growth teams.
It will help your team identify the most ideal potential customers that demonstrate the highest LTV. These are the prospects that have demonstrated a certain pattern of engagement or buying behaviors, thereby making it possible to determine future buying habits based on those projections.
The benefits of predictive analytics for the whole company
When using predictive analytics, your marketing team can find solutions to a wide variety of obstacles that get in the way of the success of your growth campaigns.
Correlation analysis to combat churn, with personalized offerings
Predictive analytics can help reduce customer churn through personalized offerings. As we all know, the ability to minimize customer churn will help reduce costs, and up the loyalty of your customer base. The way that predictive analytics can help determine who’s more likely to churn will be through the correlation analysis of data on customer profiles, transactions, and feedback. The personalized offerings come into play by using that information to offer your at-risk users a little something special to woo them back in.
Rich data to assist with forecasting, inventory management, and more
The customer behavior data that’s offered up by predictive analytics can also help your marketing team help several other departments. This is because it will provide the insights needed on how to optimize prices and improve revenue and inventory management. The sales team will also love you for it, because elements of the marketing automation data can also be used to forecast deals.
How a D2C brand increased revenue by 10 percent with predictive analytics
A major D2C brand was facing challenges when it came to pricing and inventory management in its earlier stages. Many products either sold out within hours, or barely at all, which ultimately led to lost revenue. Once they decided enough was enough, they turned to predictive analytics to get more direction, using historical sales data to predict future demand, and optimizing pricing accordingly. It was a win, and they were able to increase revenue by 10 to 13 percent across different departments! Their marketing team is continuously using this tool to understand the buying patterns of their customers and market trends, to enhance inventory management, retention, and of course growth campaigns.
We as a collective are still trying to find our way in the post-pandemic world, and marketing budgets are still a little on the tight side for most brands. More needs to be done with less. Marketers are beginning to allocate their resources to focus on targets that provide the highest ROI, and nothing provides ROI like repeat customers.
Top models of predictive analytics
Predictive analytics models go over historical data to uncover patterns and observe trends, and use those findings to to draw up predictions about future trends.
This used to be the domain of mathematicians and statisticians, but top brands are turning to predictive analytics to help solve difficult problems and uncover new opportunities—and so can you!
Some of the common uses that you can benefit from include improving operational efficiency, fraud detection, risk reduction, and the optimization of marketing campaigns.
Here’s a quick description of some of the top predictive analytics models used by sales teams:
- Forecast models handle metric value prediction by estimating the values of new data based on learnings from historical data. This is typically used to generate numerical values in historical data when there is none to be found, and it has the ability to input multiple parameters. Due to its versatility, it is used in different industries and business purposes.
- Classification models are one of the most common predictive analytics models, because they categorize information based on historical data. They can be easily retrained with new data, and can provide a broad analysis for answering questions.
- Time series model focuses on data where time is the input parameter. It uses different data points (taken from the previous year’s data) to develop a numerical metric that will predict trends within a specified period. This is useful for brands that want to see how a particular variable changes over time.
- Clustering model sorts data into different groups based on common attributes. The ability to divide data into different datasets based on specific attributes is particularly useful in certain applications, such as marketing.
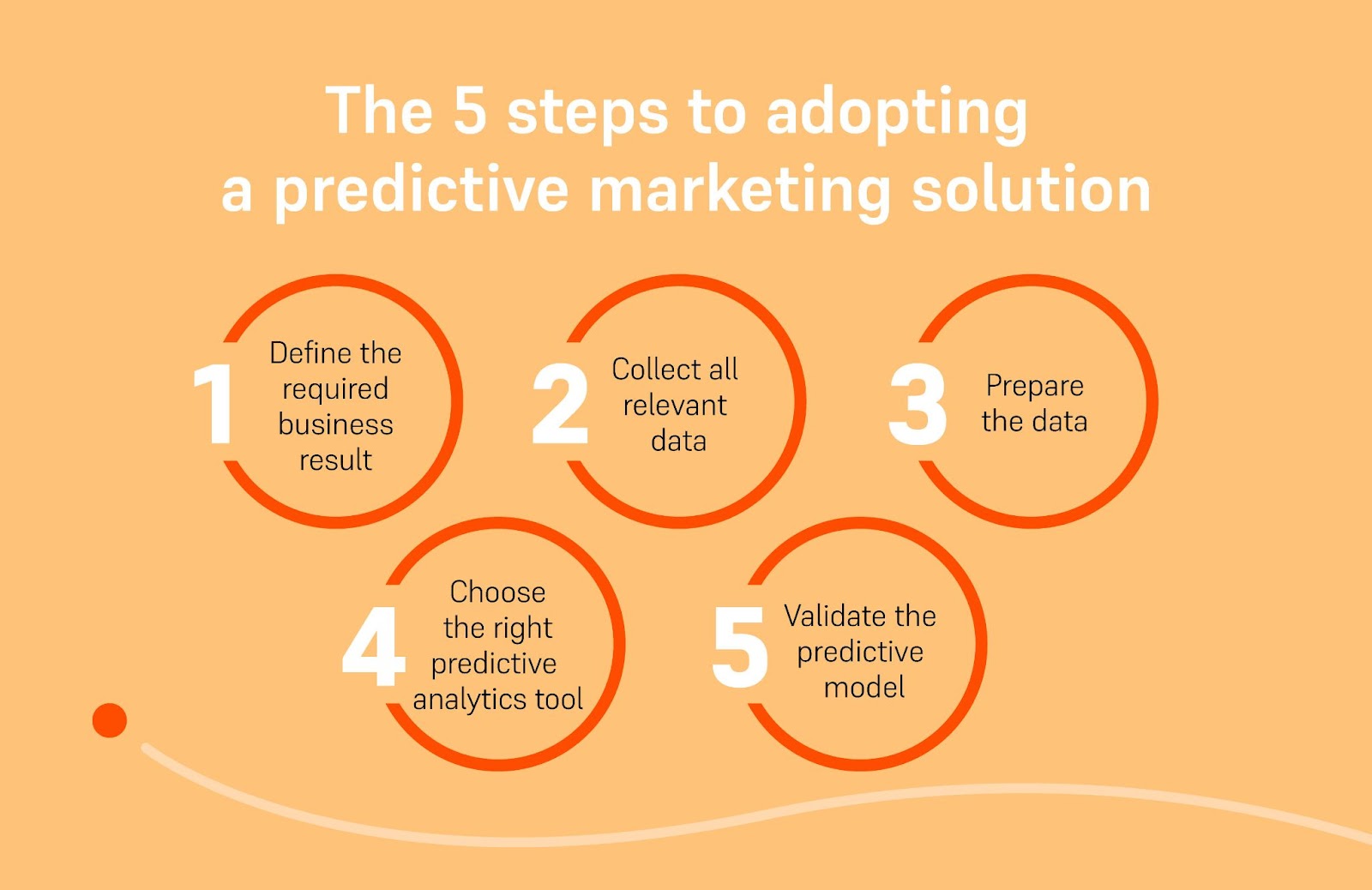
There are currently many platforms that help you streamline this process. Many of them also offer up automated features and tools that can help your engineers and data team easily apply such models in house. For example Big Query has a ML template library, which makes life easier for brands that are using GA4 (with free row data export to Big Query) and the Big Query ML library.
All in all, predictive analytics helps you learn about your past data, and also unlocks the ability to optimize the existing customer’s experience. These days, predictions can and should be used even before acquiring users, by using predictive models. There is much that can be achieved by using predictive models for UA amid oversaturated markets and increasing digital involvements. One of the main benefits is the reduction of CAC, and determining the LTV of customers. Predictive models will also help you discover lookalike audiences, to scale marketing campaigns and increase ROI. The rich marketing data that will come from all this will also help your brand deliver personalized customer experiences, upon understanding signals of intent.
How to get started with a predictive marketing solution
When it comes time for your brand to get started with a predictive marketing solution, you have to first understand what your business and technical requirements are, and work out the best solution based on those requirements. You would also need to understand which solution is the right one that fits the team, budget, scale, internal resources and more.
Everyone in the marketing team will need to be aware of the features and functionalities of the chosen solution, to understand how to capitalize on it. This needs to be seen as a marathon, and not a sprint. Together, you need to figure out the specific business results you want to achieve by using the predictive analytics tool. It would also make life easier if you’re able to feed your solution data from tools such as CRM applications, marketing tools, and other forms of software that store large amounts of data (because it gets tedious if working from spreadsheets.) Additionally, let’s not forget that it’s all about quality over quantity. The input data would need to be top tier with the right values, and as much consistency as possible. You can look into a data cleaning tool beforehand, to ensure all that, and also make necessary adjustments on duplicate records.
We addressed the top nine questions to ask before onboarding a new predictive solution for user-acquisition, in a previous blog post, because we understand that it is not an easy undertaking in a technical environment. Keep in mind that haste makes waste, so be sure to take your time in choosing the most suitable solution that will help you achieve the results you need, while requiring minimal effort on your part.
Subscribe for more
Read expert stories, interviews, reports, insights and tips for profitable growth.