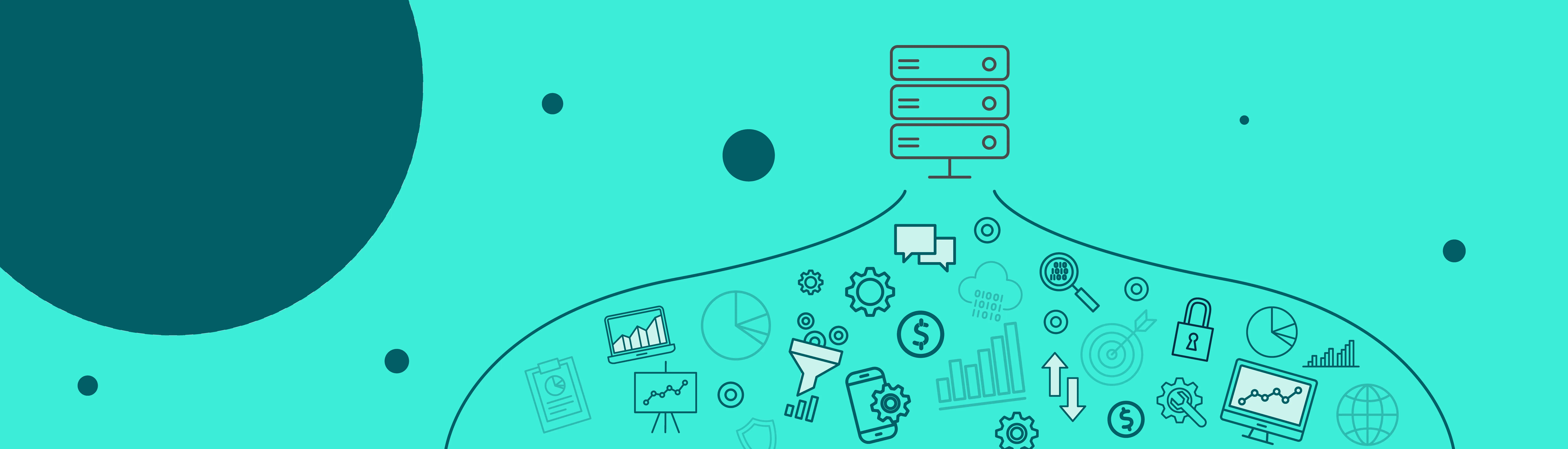
Top six ways data science helps solve growth marketing challenges
When big data gets really big, data science helps make sense of it all for growth teams, especially with the backing of AI solutions—which are quite the lifesaver (and time saver). There are many benefits to data science that make it quite the worthwhile investment. Interestingly enough—data science can fuel the efforts of many stakeholders in your organization. This includes your growth team, other branches of marketing, the product team, revenue operations, and plenty other departments.
But I know I know… you care about how it can help you and your growth team first and foremost. (We care most about that too! 😉) So on that note, here are some of the marketing challenges that data science can help solve. In many cases, AI-powered predictive platforms can provide an additional boost to bridge the gap.
Merging different data sources and types
Many brands typically store different information on their customers across different sources. For example, one source might hold static information such as gender, address, age, etc., while another has information regarding actions their customers took in the past. Extracting information from various sources where the amount of data on specific customers can vary demands major experience and tons of patience. I’m sure you can imagine how time-consuming that would be manually.
Then there are also different data types that need to be factored. For instance, there’s first-party data, which refers to the information that your brand is able to collect (and provide) from your own sources. This refers to the info about your customers that’s extracted from both online and offline sources, such as your website, app, CRM, social media, or surveys. Second-party data is typically referred to as first-party data that actually belongs to someone else. This can be extracted from other brands' websites, apps, or offline sources, such as surveys. Third-party data is the stuff you get from a total outside source, such as that acquired from a data aggregator.
Then there is zero-party data, which is becoming the most valuable data that your marketing team can use. This refers to data that is intentionally and proactively shared directly by the consumer, which you can use to determine what your customers intend to do or buy in the future. Most brands typically obtain this information in the form of questionnaires, polls, or other interactive experiences that will ultimately be a win-win for all—they get a better experience, and you get rich data.
Here’s a look at one of the ways Tide obtains zero-party data:

When you have data science infrastructure set in place, all these forms of data can basically join forces, giving you a 360 degree view of what it is your customers want, both in terms of general segments, and down to the user level. If you choose to incorporate data science into predictive marketing platforms, it becomes that much more important to bring all your data lakes together so it can be validated, cleansed, standardized, and compiled for ease in availability and optimization.
Insights and experimentation
Generally speaking, data scientists look for causal relationships-of-interest to present to growth teams. In turn, growth teams can then reverse engineer those insights into effective campaigns.
Let me explain what I mean.
For your brand, let’s assume that there appears to be a good correlation between clicks and conversion rates. If you have a data scientist on board, they can test if that is true with a regression analysis. Then someone in your growth team can collaborate with the data scientist on experiments to test which campaigns produce more clicks, leading to higher conversion rates.
Here’s what a proper data science workflow looks like, factoring the objectives of your team, leading up to the deployment and monitoring of experiments.

Of course, just because one experiment went well does not make that set-up a forever thing. Experiments have to be constant. That’s the only path to progression. With the backing of data science you will be able to perform many micro-experiments that will ultimately give you a ton of insight—all without the need for you to make drastic or sudden changes. In turn, that mitigates the risks that are inherently present when teams try something new.
Ease in segmentation and targeting specific groups
Data scientists and marketers are both all about optimization, and the root of many campaigns typically stem from segmentation, which refers to the grouping or clustering of customers into groups based on different characteristics.
Some of the perks that come from segmenting your customers include:
- Ease in determining marketing opportunities to pounce on
- Creation of tailor-made marketing initiatives as uniquely relevant for each group
- Insights that will help with product development and design elements
When using segmentation and clustering algorithms to determine which groups are worth targeting, you will be able to build a user acquisition strategy based on criteria such as payback period and future ROAS to unlock new opportunities. This includes targeting specific groups of individuals with campaigns better suited for them, such as late bloomers, which refers to users who are more likely to make a purchase later on in their user journey. They don’t necessarily purchase more but tend to stay around longer, leading to potentially higher LTV.
Data scientists and growth teams go this route because it’s a zillion times more strategic than whipping out generic campaigns that would lead to low ROI.
User-level LTV-based predictions and full funnel optimization
Imagine having the ability to use machine learning as a crystal ball... to predict each user’s future LTV, conversion, retention and loyalty. Totally possible with data science, and it works wonders on ad campaigns!
These days, LTV based optimization is no longer a matter of keep or kill campaigns or ads. The Facebook conversions API and Google’s Server-Side Tagging both allow media buyers the integration that is essential to fire back server side signals (such as LTV) in order to optimize campaigns based on them. In turn, this technology allows brands to send all sorts of new signals, such as offline conversions, and time passed from a free trial.
This ties into how data science shines when it comes to full funnel optimization. From a traditional standpoint, marketing campaigns have mostly been focused on awareness, acquisition, and activation. Data science turns that up many notches, allowing marketers to get all the way down to revenue, retention, and referral. Instead of focusing just on the first conversion, your team can use data science insights to target longer-lasting relationships with customers.
We might take all this for granted now, but bear in mind that in the past, all brands, regardless of their size, had to spend a considerable amount of money to hire research firms to gain some type of understanding of the sentiments of people when it came to their brand’s products and/or marketing campaigns. Not anymore! These days, data scientists play the role of the research firm and then some, because you know the insights from data science are all the real deal, without a shadow of doubt.

Greater productivity, with the added bonus of hyper-personalization
AI-powered marketing solutions allow your brand to automate many repetitive tasks, which will lead to an increase in productivity. “More than half (51%) of decision making acknowledges that cost reduction is the first area AI is offering,” says Joe McKendrick of Forbes.
The automation element of repetitive tasks will be far from the blanket messaging that’s like “Dear Customer…” With the advances in machine learning, AI is unlocking the potential of hyper-personalization through personalized product recommendations, intelligent content recommendations, and customer support suggestions. Additionally, by looking at insights given to you by customer persona data, you can perform various clustering analyses to see what else they are likely to buy and what price they are likely to buy it at. These insights let you know exactly what your customer is looking for both from your current collection and give you data to develop new products they might be interested in—a godsend for eCommerce brands.
And it can get even deeper than that, with customer response predictions. Imagine having a supervised model that can predict whether a person will respond to a marketing campaign or not. That’ll eliminate a TON of guesswork.
This kind of ties in with how Netflix uses data science. They work to keep their customers coming back for more, by recommending new films and series’ based on the viewing history of users with similar interests. Though the first-hand effect for the user is enriching, helpful, and personal, the ultimate goal is to keep the user subscribed month after month.
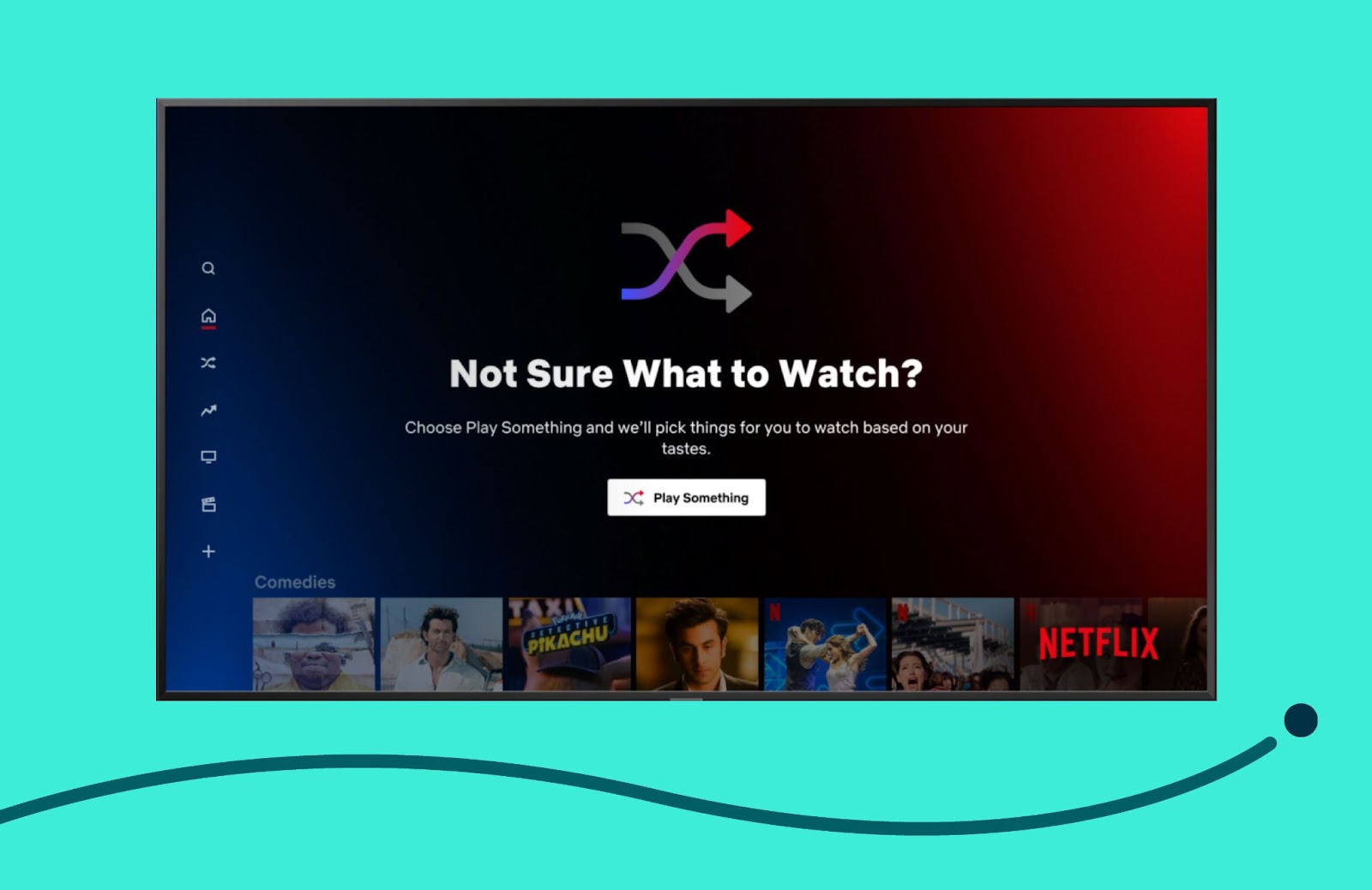
Data science also gives you the power to communicate with your customers quickly based on real-time data. For example, your team might want to target customers who have a bad experience who are at risk of churn. Data science allows you to find customers who fit the mold and market to them immediately. This helps brands improve their customers’ experience by further personalizing content.
Increase in profitability, and reduction in costs
Data science, when done right, ensures that every penny of your business’s marketing budget is spent in a useful way that maximizes profits.
When paired with AI, data science can help your brand enhance profitability and reduce costs in many forms, such as planning your media budget for reaching your KPIs with AI. As stated on Forbes, once an organization has calculated what it hopes to save by implementing AI, only then should it begin to consider how much to invest. Many organizations struggle with predicting the break-even point for AI deployments. By allowing cost-savings to dictate the initial AI investment, companies can begin to estimate when the break-even point should be reached.
Data science: a lifesaver for any and every team
The benefits that stem from incorporating data science will quickly outweigh any initial hiring or set-up costs.
These benefits go beyond everything I listed out in this post. You can also use it to learn from customer feedback, turn more leads into conversions with cross- and up-selling, and refine your digital advertising.
At this point, it’s downright silly and needless to waste less time and money on trial-and-error marketing plans. Now more than ever, growth teams cannot afford to not incorporate data science into their marketing plan, and that is because data science is an indispensable resource that’ll elevate your brand to new heights.
Subscribe for more
Read expert stories, interviews, reports, insights and tips for profitable growth.