
From lookalikes to do-alikes: How to push ad networks to find high LTV users
What are your thoughts on Facebook’s Lookalike Audiences? Personally, I’d say it’s a pretty cool feature for growth teams to be able to target audiences based on their custom audiences, such as people on email lists, and website visitors.
Lookalikes are the starting point for a Facebook campaign, which then needs a lighthouse, or better yet, a laser beam, to track the combination of creative + placement + audience + product fit. Of course, the best approach is to start and look in a relevant area, but then you should augment this with LTV signaling, to further narrow down your performance optimization and really put it on hyperdrive.
Simply put, we can’t expect Lookalike Audiences to do all the work. Otherwise, we'd all probably be optimizing solely on installs and signups.
{{banner}}
Quick rundown on how lookalikes work
So like… let’s say you’re a subscription brand with an email list of 10K premium subscribers. Wouldn’t you want more purchasers like them, to reel in more profitability? This is where lookalike audiences come in. You would upload that list of subscribers, and I’d imagine Facebook would be able to match up more than half of those emails to those associated with their accounts. So what will Facebook do? They will use that sweet data thay have on the attributes of the matched accounts, and show your ads to other users, with similar attributes.
It’s quite the timesaver for marketing teams, because it eliminates the guesswork that is associated with ad targeting. And it is all made much merrier when creating lookalike lists based on high LTV users.
Actually, all growth campaigns become significantly more impactful when optimizing for high LTV events. Allow me to explain why.
Facebook undoubtedly knows a lot about its users. The network can look at your custom list of people (called a source, or seed audience), then match them with their database of Facebook profiles, and target users with similar interests and demographics. Facebook can reach the top 1%-10% most similar users by chosen location (state or city), based on the uploaded list (can be any list of users with email addresses/phone numbers).
Choosing the right seed audience for the lookalike audience is the first step towards targeting your most desired audience. You can create it using data from:
- Website visitors
- Customer information lists (such as wish list submitters)
- App activity users
- Platform engagement (video viewers, page likes, etc)
- Offline activities (such as local store customers)
It is important to bear in mind that all of these serve as the starting point for the algorithm, before it aims precisely on the most qualified users for desired actions. Lookalikes are the starting point, and the actual campaign traffic lower-funnel performance (on the chosen ad-set optimization goal, e.g. signup, purchase, newsletter registration) is the feedback loop it uses to hone in on that lower-funnel metric.
From high LTV seed, to high LTV optimization
The usage of lookalike audiences has proven to be helpful for many brands of all industries and sizes. Metaphorically speaking, it can be seen as a really bright street lamp that is casting light on a large group of people (who happen to have similar attributes to those on the list originally provided.)
So if you have a list of 200,000 of your highest value users, and you create a new campaign using this list as a lookalike seed, Facebook will target millions of additional users that are similar to your list. The similarities will exist because of the seed, but it also varies. There are even settings that allow you to control just how similar your audience would be, starting from size options of the audience.
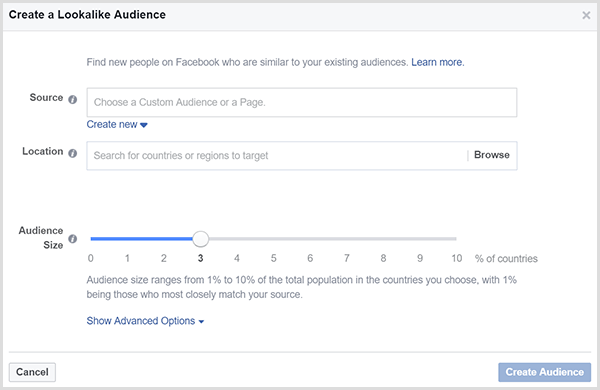
Using the Facebook algorithm to find the most valuable users is the next step after choosing your base audience. You can accomplish this by optimizing campaigns with the right optimization events.
By selecting the event, the Facebook system learns how to show ads to the people who are most likely to get the result you are optimizing for, within the selected attribution setting.
In campaign types like Automated App Ads (which is almost completely automated without any targeting options), it is extremely relevant. Ad delivery can be optimized by showing your ads to people who are the most likely to perform specific actions that are valuable for your business.
All this becomes more elevated when optimizing campaigns for high LTV.
If lookalikes are like wide street lamps, I’d say that using a high LTV signal/event is like using a laser beam. Not an itty-bitty laser beam—I’m talking about something huge and practically military-grade. Here’s how that works.
When optimizing for high LTV events on any platform, you're sending them very focused signals on which users are high LTV for your brand, and empowering them to target more users just like those by learning the signal, and optimizing your campaign for it.

So if in the previous example FB targeted millions of users based on the lookalike list when optimizing using a high LTV signal, Facebook will target a narrower group of users, which is more accurate. Less is definitely more in this case, because it focuses more on the quality of users instead of the quantity. You’re zeroing in on the crème de la crème.
There are plenty of other benefits that coincide with using LTV events for optimization. For instance, since the signals are sent and used in real-time, the data is fresher. Additionally, the signal reflects the user’s reaction to a specific campaign/creative, as opposed to a lookalike list, which only provides the notion of a user being a good user. Who wouldn’t want to attract users that are far more inclined to get the ball rolling, and keep on rolling for the long-term.
LTV-based optimization: What you should know
LTV-based optimization refers to using LTV data to be able send signals to ad networks, and use them as optimization goals. When building out a growth and acquisition strategy, LTV-based optimization plays a direct role in long-term profitability. These campaigns are different from most other UA campaigns by nature, and it is important for teams to understand what to expect (in terms of rollout) before embarking on this approach.
LTV-based optimization falls under the predictive marketing umbrella (using data to forecast future customer behavior), and can be done in-house leveraging an existing LTV model.
We actually created a useful guide that delves into how LTV data, when used as a predictive metric, translates to empowerment for growth teams as it helps bring out the full potential of user acquisition campaigns. This post, which links to the guide, talks more about that.
Of course, not all teams have the time or resources to stay on top of the LTV optimization process, because there are many technicalities involved. For starters, there’s a learning period for the ad networks to learn the new signal that has been given. Other metrics may temporarily fluctuate during that period. There are also some trends that can be expected. But once all is said and done, the campaigns that optimize for LTV end up showing exponentially higher ROI. Brands that need a helping hand in navigating through this process best team up with a predictive marketing partner, to help ensure that there aren’t any costly missteps being made along the way.
It’s safe to say that at the end of the day, it all boils down to what your goals are with each campaign. Sometimes, you might actually need the wide coverage that’s offered by the street light. But when you’re looking to achieve maximum impact and profitability with customer/user acquisition, activation, retention, and upsell in mind—you would be better off utilizing LTV signals for maximum optimization and results that last.

Subscribe for more
Read expert stories, interviews, reports, insights and tips for profitable growth.